Non-Parametric Tests and Their Classifications
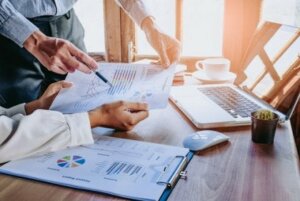
Non-parametric tests or techniques encompass a series of statistical tests that lack assumptions about the law of probability that follows the population a sample has been drawn from. These tests apply when researchers don’t know if the population the sample came from is normal or approximately normal.
Non-parametric tests are used often because many variables don’t follow the conditions of parametricity. Those variables are the use of continuous quantitative variables, normal distribution of the samples, similar variants, and balanced samples.
When the above requirements aren’t met or there are doubts about whether they meet the requirements, statisticians use non-parametric or free distribution tests. Non-parametric tests share these traits:
- Researchers use them less than they should (they aren’t as well-known).
- They’re applicable to hierarchized data.
- You can use them when two series of observations come from distinct populations (populations in which the variable isn’t equally distributed).
- They’re the only realistic alternative when the sample size is small.
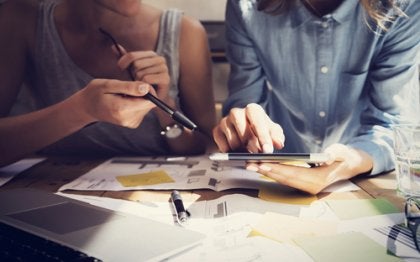
Classification of non-parametric tests
There’s a lack of consensus on the classification of non-parametric tests when it comes to grouping them together. Authors Berlanga and Rubio (2012) wrote a summary of the main non-parametric tests.
Non-parametric tests of one sample
Pearson’s chi-squared test
This test is useful when a researcher wants to analyze the relationship between two quantitative variables. It’s also helpful to evaluate to what extent the collected data in a categorical variable (empirical distribution) adjusts or not (is similar or not) to a determined theoretical distribution (uniform, binomial, multinomial, etc.).
Binomial test
This test allows the tester to find out if a dichotomic variable follows or doesn’t follow a determined probability model. As a result, it makes it possible to contrast the hypothesis that the observed proportion of correct answers adjusts to the theoretical proportion in a binomial distribution.
Runs test
This is a test that allows the researcher to determine if the number of runs (R) observed in a sample of size (n) is big enough or small enough to be able to reject the independence (or randomness) hypothesis among the observations.
A run is a sequence of observations of a single attribute or quality. The presence of more or fewer runs than can be attributed to randomness in a series of data could be an indicator that there’s an important variable that’s conditioning the results and you aren’t taking into account.
Kolmogorov-Smirnov (K-S) test
This test is useful for contrasting the null hypothesis that the distribution of a variable adjusts to a determined theoretical probability distribution (normal, exponential, or Poisson). That the distribution of data points adjust or not to a determined distribution helps determine which data analysis techniques are best for the specific situation.
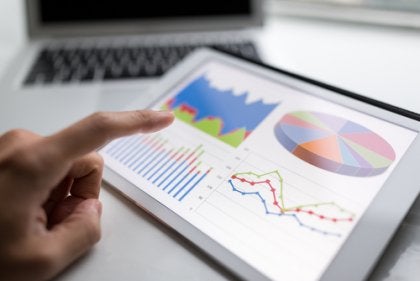
Non-parametric tests for two related samples
McNemar test
Statisticians use the McNemar test to contrast hypotheses about the equality of proportions. It’s useful when there’s a situation in which the measures of each subject repeat themselves. Thus, the data returns the answer of each one of those twice: once before and once after a specific event.
Sign Test
This allows researchers to contrast the equality hypothesis between two population medians. They can also use it to find out if one variable tends to be greater than another. It’s also useful for testing the trends that follow a series of positive variables.
Wilcoxon Test
The Wilcoxon test makes it possible to contrast the equality hypothesis between two population medians.
Non-parametric tests for related K-samples
Friedman test
This is an extension of the Wilcoxon test. Researchers use it to include registered data from more than two periods of time or groups of three or more subjects. In these tests, one subject from each group has been randomly assigned to one of the three or more conditions.
Cochran test
This is identical to the last, but it applies when all the answers are binary. Cochran’s Q also approves the hypothesis that dichotomous variables that are related among themselves have the same average.
Kendall’s W
This test has the same instructions as the Friedman test. Its use for research, however, has been primarily to find out the concordance between ranges.
Non-parametric tests for two independent samples
Mann-Whitney U
This is equivalent to the Wilcoxon range sum test and also the Kruskal-Wallis two-group test.
Kolmogorov-Smirnov Test
Researchers use this test to contrast the hypothesis that two samples come from the same population.
Wald-Wolfowitz Run test
This contrasts if two samples with independent data come from populations with the same distribution.
Moses test of extreme reaction
This tests to see if there are differences in the degree of dispersion or variability in two distributions. It focuses on the distribution of the control group. It’s also a measure to find out how many extreme values of the experimental group influence the distribution when combined with the control group.
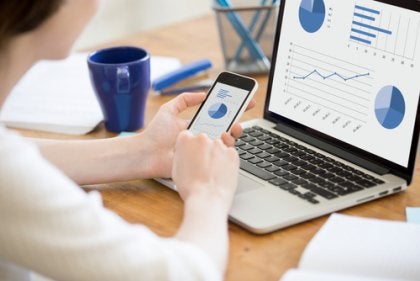
Non-parametric tests for independent K-samples
Median tests
These tests contrast differences between two or more groups in relation to the median. They don’t use averages, either because they don’t meet the conditions of normality, or because the variable is discrete quantitative. It’s similar to the Chi-squared test.
Jonckheere-Terpstra test
This is the most effective test if you want to analyze the ascending or descending order of K populations from which the sample was drawn.
Kruskal-Wallis H tests
Lastly, the Kruskal-Wallis H test is an extension of the Mann-Whitney U test and represents an excellent alternative to the ANOVA test of a factor.
In conclusion, statisticians and researchers use these tests when the distribution of data isn’t normal. You can also use these whenever you have data that isn’t on a reasonable scale, or, if it is, you have doubts about whether or not the distribution of variables follows the normal curve. While it’s true that a lot of parametric tests are fairly good at handling data that violates the suppositions, there are better ones out there, so why not use them?
All cited sources were thoroughly reviewed by our team to ensure their quality, reliability, currency, and validity. The bibliography of this article was considered reliable and of academic or scientific accuracy.
-
Berlanga-Silvente, V., & Rubio-Hurtado, M. J. (2012). Classificació de proves no paramètriques. Com aplicar-les en SPSS. REIRE. Revista d’Innovació i Recerca en Educació, 5(2), 101-113.
This text is provided for informational purposes only and does not replace consultation with a professional. If in doubt, consult your specialist.