Descriptive Statistics in Psychology
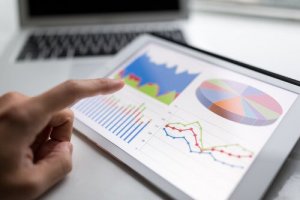
Statistics is the branch of mathematics that studies variability, as well as the process that generates it by following the laws of probability. It’s necessary both to do research and to understand how it’s currently being conducted beyond the conclusions of any study. Thus, knowledge about the branch of descriptive statistics will allow you to know, to a large extent, the quality of a study. Therefore, the degree of reliability its conclusions deserve.
Descriptive statistics, meanwhile, is that part of statistics responsible for collecting, presenting, and characterizing a set of data. In other words, descriptive statistics intend to know what happened, compared to inferential statistics that try to predict what’ll happen in the future under a set of conditions.
For example, these conditions are usually specified using variables such as age, climate, or degree of anxiety. Thus, descriptive statistics in psychology have the goal of usefully summarizing what happened in a determined study for both the researchers and readers.
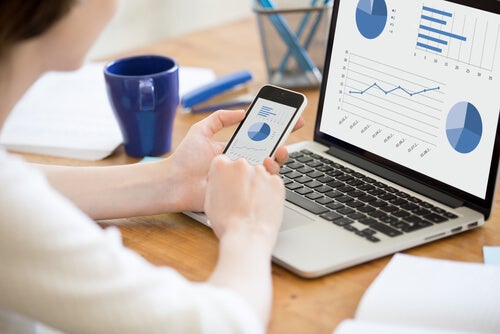
Variables in descriptive statistics
As we mentioned above, variables are one of the central axes of descriptive statistics, and of non-descriptive statistics as well. A variable encompasses a set of values. Depending on their value, we have:
- Quantitative variables. These can have numerical value (for example, age, price of a product, or annual income).
- Categorical or qualitative variables. You can’t measure them numerically (such as sex, nationality, or skin color) or scale them directly.
You can also classify variables into:
- One-dimensional variables. These only collect information about a characteristic of a population. For example, the height of students in a school.
- Two-dimensional variables. They collect information on two characteristics of the population. For example, the height and age of students in a school.
- Multidimensional variables. They collect information about three or more characteristics of a population. For example, the height, weight, and age of students in a school.
Thus, the data (numbers or measurements collected from the observation) can be of two types:
- Discrete data. Numerical responses that arise from a counting process.
- Continuous data. Numerical responses that arise from a measurement process.
Measurement scales in descriptive statistics
Measurement is the process of linking abstract concepts with empirical indicators. The result of measuring is a measurement.
There are four possible measurement scales, which you can use to aid you in the classification of variables. In this sense, the reliability and validity properties are very important in descriptive statistics. This is because they tell you about the quality of the measurement. Also, because what’s the use of wrong data collected from the beginning?
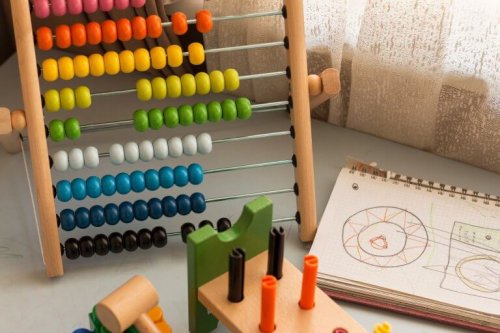
Nominal scale
On this scale, one assigns numbers to categories that don’t need an order (you can’t say that one category is greater than another). Furthermore, these categories are mutually exclusive. An example of this may be gender or color. Thus, the option you choose excludes some of the rest.
You can assign this scale to qualitative or categorical variables.
Ordinal scale
Here, you establish categories with two or more levels and they imply an order among themselves. As in the previous scale, these are mutually exclusive categories as well. But now you can put the values of the variables in order. For example, the possible answers to a questionnaire:
- Strongly disagree
- Somewhat disagree
- Indifferent
- Agree
- Completely agree
You can code these response options with numbers that suggest a preset order, ranging from one to five. However, you can’t know, unless you use advanced statistical procedures and try to estimate the distance between two categories. Thus, you can speak of the fact that the object of the investigation has more or less of something but can’t speak of how much more of that something (intelligence, memory, anxiety, etc.).
You can also assign this scale to qualitative variables.
Interval scale
On this scale, you can quantify the distance between the values. The interval measurement also has the characteristics of the two previous measurements. Thus, it establishes the distance between one measurement and another.
You can apply the interval scale to continuous variables. However, absolute zero isn’t possible on it. A clear example of this type of measurement is a thermometer. Note that a zero degree reading doesn’t imply an absence of temperature.
You can apply this scale in quantitative variables.
Ratio scale
Finally, this scale reflects the characteristics of the previous ones. Determine the exact distance between intervals in a category. In addition, it has an absolute zero point where the characteristic or attribute you’re measuring doesn’t exist. For example, the number of children: zero children means the absence of children.
You can apply this scale in quantitative variables.
Frequencies in descriptive statistics
A frequency distribution is a list of the possible values (or intervals) that a variable takes, along with the number of observations for each value.
- The absolute frequency records the number of times that a certain value appears between the observations.
- The relative frequency records the proportion or percentage of occurrence of a certain value of the observations.
This frequency distribution is usually represented by tables. Thus, it must include all the possible values of a variable. In addition, you must indicate the total number of observations (n). When you have a large amount of data categories and some of them with very low frequencies, then you must group them in intervals.
Indicators
Finally, indicators in statistics are used to describe a data set using a number. Thus, this number summarizes a characteristic of the distribution of the analyzed data. Some of these indicators are:
Indicators of central tendency
- Mean or average
- Mode
- Median
Dispersion indicators
- Variance
- Minimum and maximum
- Rank
- Interquartile range (IQR)
With the help of these concepts, descriptive statistics is in charge of debugging, organizing, and calculating statistics and data representations to offer the researcher and the scientific community a complete map of what happened in their study.
All cited sources were thoroughly reviewed by our team to ensure their quality, reliability, currency, and validity. The bibliography of this article was considered reliable and of academic or scientific accuracy.
-
de Datos, A. E. (1983). Estadística Descriptiva.
-
Fernández, S. F., Sánchez, J. M. C., Córdoba, A., Cordero, J. M., & Largo, A. C. (2002). Estadística descriptiva. Esic Editorial.
-
Parra, J. M. (1995). ESTADÍSTICA DESCRIPTIVA E INFERENCIAL I.
This text is provided for informational purposes only and does not replace consultation with a professional. If in doubt, consult your specialist.